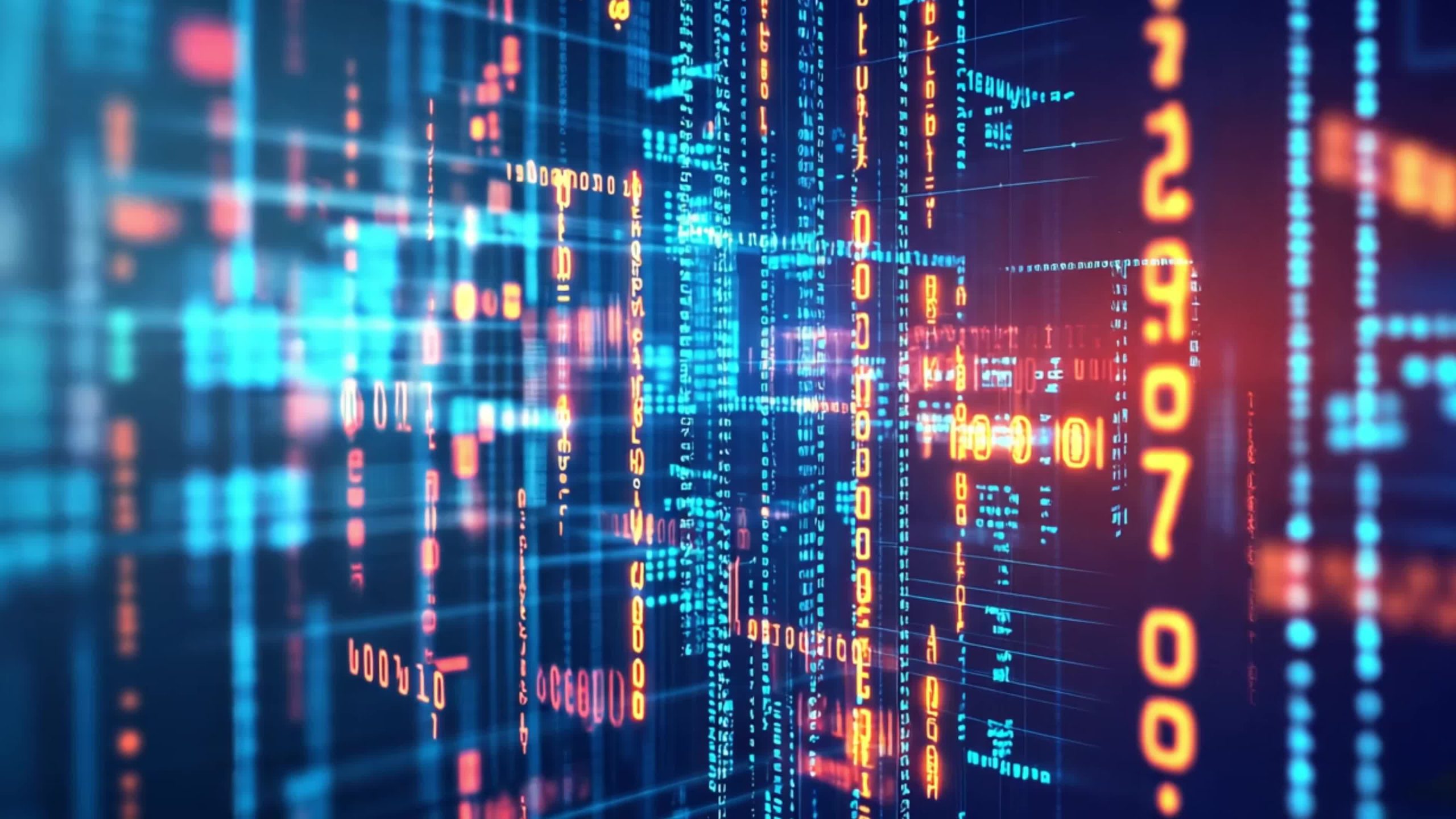
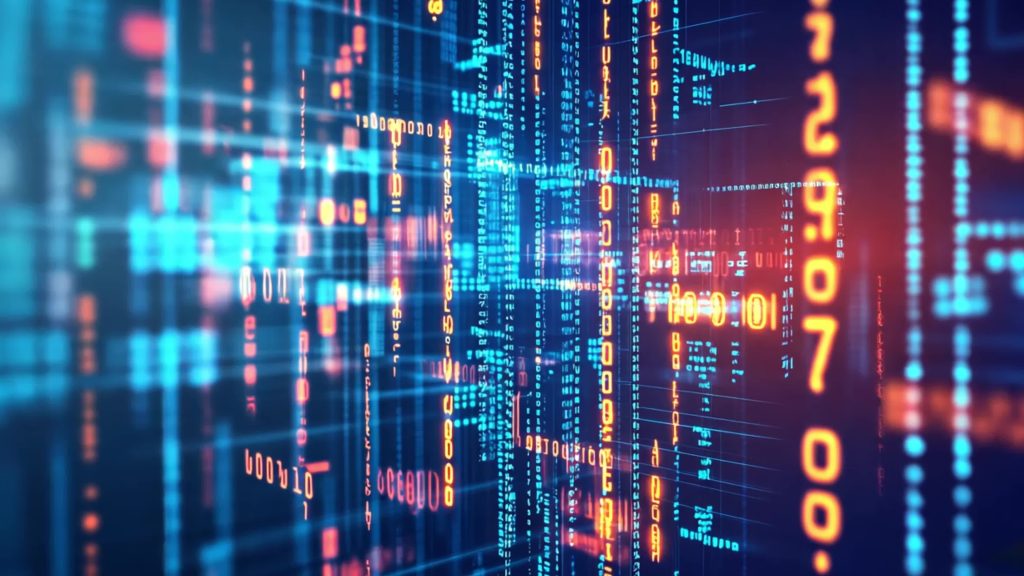
In 2010, media monitoring giant Cision faced the ultimate challenge of data integration – unifying globally dispersed media databases, news clips, and customer legacy systems onto the SaaS platform CisionPoint. Its technical team found that data cleaning and mapping in the European market alone require hundreds of hours of manual operation, and traditional ETL tools are difficult to handle unstructured data encoding. The data quality tool of DataMlux helped Cision improve the accuracy of postal code recognition to 98% within three months through address correction modules and flexible web service interfaces, and established a dynamic data pipeline to compress the real-time synchronization delay between North American and European systems from 48 hours to 2 hours.
This case reveals the core contradiction of digital transformation: enterprises need to maintain the stability of Unix legacy systems while also adapting to real-time collaboration needs in the cloud. The solution of DataMlux uses a pluggable architecture to ensure data traceability while allowing development teams to customize cleaning rules using Python, reducing reliance on dedicated data engineers. Currently, similar technologies are being applied to AI training data governance, such as automatically annotating metadata anomalies in video streams.