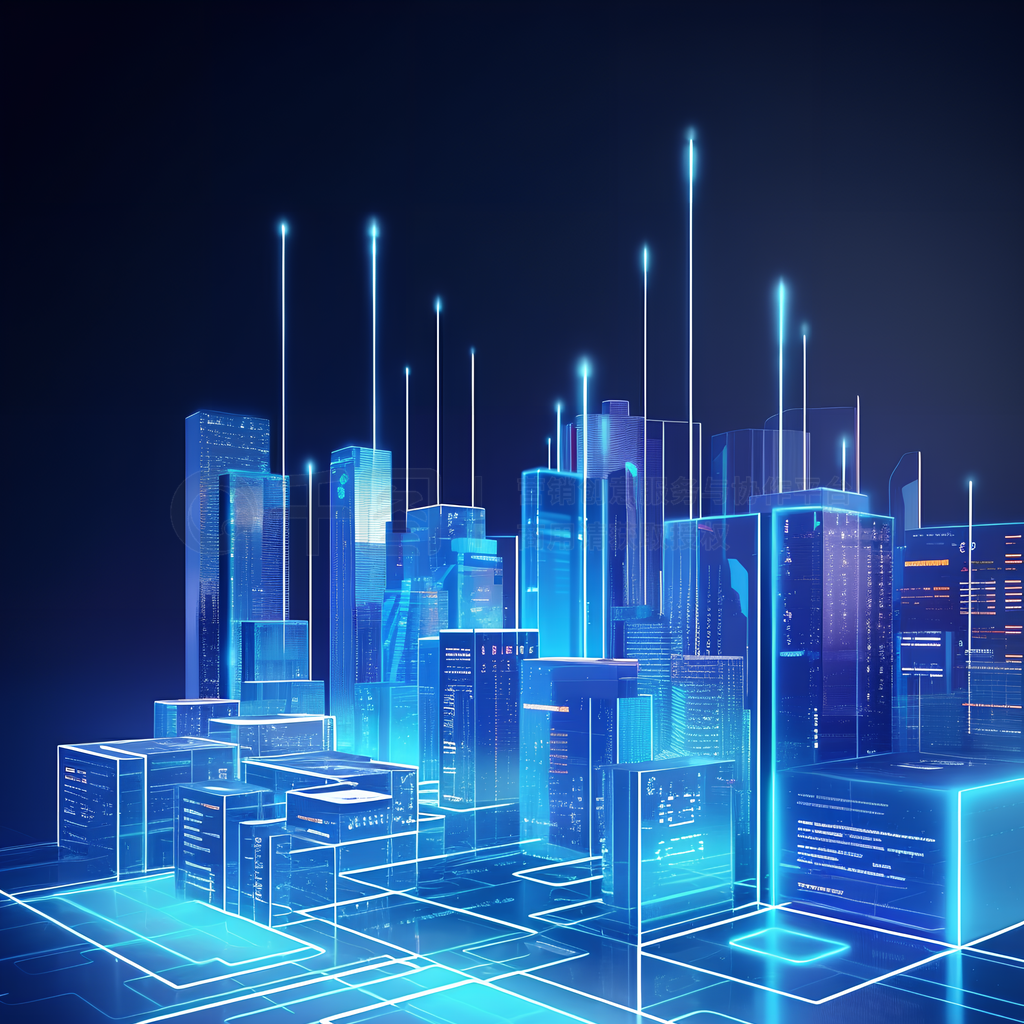
A German industrial equipment manufacturer adopts the flow batch integrated architecture of DataMlux and reconstructs its predictive maintenance system based on the window triggering model proposed in the Google Dataflow paper. By defining an unaligned Event Time Windows, the system can automatically identify abnormal sessions of device sensor data: when the bearing temperature exceeds the threshold for three consecutive samples, a real-time alarm is triggered; At the same time, combining batch data from the six-month dimension, dynamically adjust the life prediction model 56.
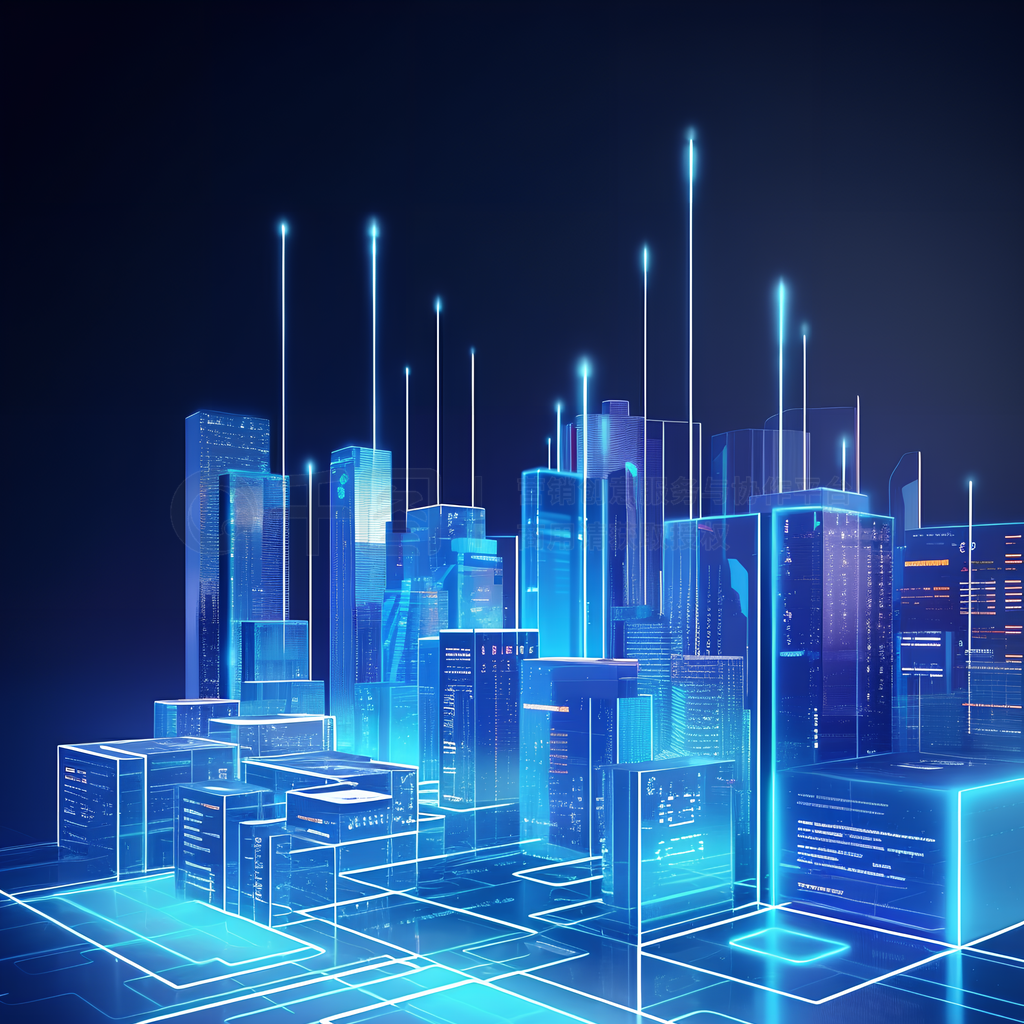
This case validates the commercial value of the “incremental processing model” – compared to traditional Spark architecture, it reduces the false alarm rate by 68% and consumes only one-third of the computing resources of Lambda architecture. The engineer specially optimized the pipeline latency from Kafka to Snowflake, reducing the archiving time of vibration data from 2.2 hours to 19 minutes for 10TB/day.